Plenary Talks
Tue, 24 May, 01:50 - 03:00 UTC
Closed-Loop Data Transcription via Minimaxing Rate Reduction
Chair: C.-C Jay Kuo, University of Southern California, USA
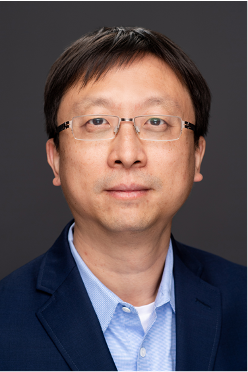
Abstract
This work proposes a new computational framework for learning an explicit generative model for real-world datasets. More specifically, we propose to learn a closed-loop transcription between a multi-class multi-dimensional data distribution and a linear discriminative representation (LDR) in the feature space that consists of multiple independent linear subspaces. We argue that the optimal encoding and decoding mappings sought can be formulated as the equilibrium point of a two-player minimax game between the encoder and decoder. A natural utility function for this game is the so-called rate reduction, a simple information-theoretic measure for distances between mixtures of subspace-like Gaussians in the feature space. Our formulation draws inspiration from closed-loop error feedback from control systems and avoids expensive evaluating and minimizing approximated distances between arbitrary distributions in either the data space or the feature space. To a large extent, this new formulation unifies the concepts and benefits of Auto-Encoding and GAN and naturally extends them to the settings of learning a both discriminative and generative representation for multi-class and multi-dimensional real-world data. Our extensive experiments on many benchmark imagery datasets demonstrate tremendous potential of this new closed-loop formulation: we notice that the so learned features of different classes are explicitly mapped onto approximately independent principal subspaces in the feature space; and diverse visual attributes within each class are modeled by the independent principal components within each subspace. This work opens many deep mathematical problems regarding learning submanifolds in high-dimensional spaces as well as suggests potential computational mechanisms about how memory can be formed through a purely internal closed-loop process.
This is joint work with Xili Dai, Shengbang Tong, Mingyang Li, Ziyang Wu, Kwan Ho Ryan Chan, Pengyuan Zhai, Yaodong Yu, Michael Psenka, Xiaojun Yuan, Heung-Yeung Shum. A related paper can be found at: https://arxiv.org/abs/2111.06636
Biography
Yi Ma is a Professor at the Department of Electrical Engineering and Computer Sciences at the University of California, Berkeley. His research interests include computer vision, high-dimensional data analysis, and intelligent systems. Yi received his Bachelor’s degrees in Automation and Applied Mathematics from Tsinghua University in 1995, two Masters degrees in EECS and Mathematics in 1997, and a PhD degree in EECS from UC Berkeley in 2000. He has been on the faculty of UIUC ECE from 2000 to 2011, the principal researcher and manager of the Visual Computing group of Microsoft Research Asia from 2009 to 2014, and the Executive Dean of the School of Information Science and Technology of ShanghaiTech University from 2014 to 2017. He then joined the faculty of UC Berkeley EECS in 2018. He has published about 120 conference papers, 60 journal papers, and three textbooks in computer vision, generalized principal component analysis, and high-dimensional data analysis. He received the NSF Career award in 2004 and the ONR Young Investigator award in 2005. He also received the David Marr prize in computer vision from ICCV 1999 and best paper awards from ECCV 2004 and ACCV 2009. He has served as the Program Chair for ICCV 2013 and the General Chair for ICCV 2015. He is a Fellow of IEEE, ACM, and SIAM.
Wed, 25 May, 01:50 - 03:00 UTC
Deep Neural Networks: A Nonparametric Bayesian View with Local Competition
Chair: Athina Petropulu, Rutgers, The State University of New Jersey, USA
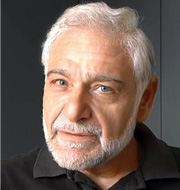
Norbert Wiener Award Recipient
Abstract
In this talk, a fully probabilistic approach to the design and training of deep neural networks will be presented. The framework is that of the nonparametric Bayesian learning. Both fully connected as well as convolutional networks (CNNs) will be discussed. The structure of the networks is not a-priori chosen. Adopting nonparametric priors for infinite binary matrices, such as the Indian Buffet Process (IBP), the number of weights as well as the number of nodes or number of kernels (in CNN) are estimated via the resulting posterior distributions. The training evolves around variational Bayesian arguments.
Besides the probabilistic arguments that are followed for the inference of the involved parameters, the nonlinearities used are neither squashing functions not rectified linear units (ReLU), which are typically used in the standard networks. Instead, inspired by neuroscientific findings, the nonlinearities comprise units of probabilistically competing linear neurons, in line with what is known as the local winner-take-all (LTWA) strategy. In each node, only one neuron fires to provide the output. Thus, neurons, in each node, are laterally (same layer) related and only one “survives”; yet, this takes place in a probabilistic context based on an underlying distribution that relates the neurons of the respective node. Such rationale mimics closer the way that the neurons in our brain co-operate.
The experiments, over a number of standard data sets, verify that highly efficient (compressed) structures are obtained in terms of the number of nodes, weights and kernels as well as in terms of bit precision requirements at no sacrifice to performance, compared to previously published state of the art research. Besides efficient modelling, such networks turn out to exhibit much higher resilience to attacks by adversarial examples, as it is demonstrated by extensive experiments and substantiated by some theoretical arguments.
The presentation mainly focuses on the concepts and the rationale behind the methodology and less on the mathematical details.
Biography
Sergios Theodoridis is currently Professor Emeritus of Signal Processing and Machine Learning in the Department of Informatics and Telecommunications of the National and Kapodistrian University of Athens, Greece and he is Distinguished Professor in Aalborg University, Denmark. His research areas lie in the cross section of Signal Processing and Machine Learning. He is the author of the book “Machine Learning: A Bayesian and Optimization Perspective” Academic Press, 2nd Ed, 2020, the co-author of the book “Pattern Recognition”, Academic Press, 4th ed. 2009, and the co-author of the book “Introduction to Pattern Recognition: A MATLAB Approach”, Academic Press, 2010. He is the co-author of seven papers that have received Best Paper Awards including the 2014 IEEE Signal Processing Magazine Best Paper Award and the 2009 IEEE Computational Intelligence Society Transactions on Neural Networks Outstanding Paper Award. He is the recipient of the 2021 IEEE SP Society Norbert Wiener Award, the 2017 EURASIP Athanasios Papoulis Award, the 2014 IEEE Signal Processing Society Education Award and the 2014 EURASIP Meritorious Service Award. He has served as Vice President IEEE Signal Processing Society, and as President of the European Association for Signal Processing (EURASIP).
He is Fellow of IET, a Corresponding Fellow of the Royal Society of Edinburgh (RSE), a Fellow of EURASIP and a Life Fellow of IEEE.
Thu, 26 May, 01:50 - 03:00 UTC
Biosignal Processing for Adaptive Cognitive Systems
Chair: Douglas O'Shaughnessy, Énergie Matériaux Télécommunications Research Centre, Canada
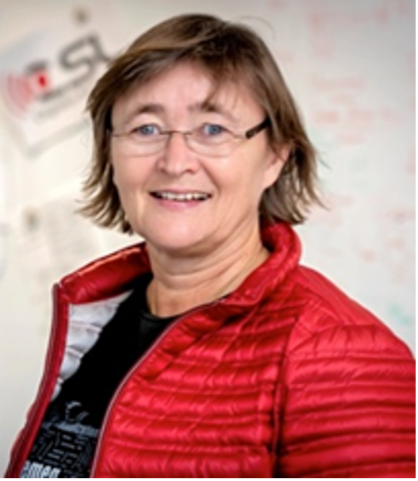
Abstract
In my talk, I will describe technical cognitive systems that automatically adapt to users’ needs by interpreting their biosignals. Human behavior includes physical, mental, and social actions that emit a range of biosignals which can be captured by a variety of sensors. The processing and interpretation of such biosignals provides an inside perspective on human physical and mental activities, complementing the traditional approach of merely observing human behavior. As great strides have been made in recent years in integrating sensor technologies into ubiquitous devices and in machine learning methods for processing and learning from data, I argue that the time has come to harness the full spectrum of biosignals to understand user needs. I will present illustrative cases ranging from silent and imagined speech interfaces that convert myographic and neural signals directly into audible speech, to interpretation of human attention and decision making from multimodal biosignals.
Biography
Tanja Schultz is Professor for Cognitive Systems of the Faculty of Mathematics & Computer Science at the University of Bremen, Germany and adjunct Research Professor of the Language Technologies Institute at Carnegie Mellon, PA USA. She received the diploma and doctoral degrees in Informatics from University of Karlsruhe and a Master degree in Mathematics and Sport Sciences from Heidelberg University, both in Germany. In 2007, she founded the Cognitive Systems Lab (CSL) and serves as Director since then. She is the spokesperson of the University Bremen high-profile area “Minds, Media, Machines” and helped establish the Leibniz Science Campus on Digital Public Health in 2019 for which she serves on the board of directors.
Professor Schultz is a recognized scholar in the field of multilingual speech recognition and cognitive technical systems, where she combines machine learning methods with innovations in biosignal processing to create technologies such as in “Silent Speech Communication” and “Brain-to-Speech”. She is a Fellow of the IEEE, elected in 2020 “for contributions to multilingual speech recognition and biosignal processing”; a Fellow of the International Speech Communication Association, elected in 2016 “for contributions to multilingual speech recognition and biosignal processing for human-machine interaction”; a Fellow of the European Academy of Science and Arts (2017), and a Fellow of the Asian-Pacific Artificial Intelligence Association (2021). Her recent awards include the Google Faculty Research Award (2020 and 2013), the ISCA/EURASIP Best Journal Paper Award (2015 and 2001), the Otto Haxel Award (2013), and the Research Award for Technical Communication from the Alcatel-Lucent Award (2012) “for her overall scientific work in the interaction of human and technology in communication systems”.
Fri, 27 May, 01:50 - 03:00 UTC
What Is Next in Signal Processing for MIMO Communication?
Chair: Sumei Sun, Institute for Infocomm Research, A*STAR, Singapore
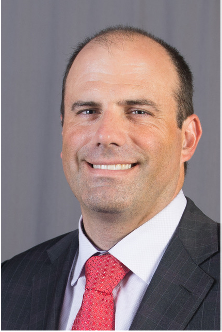
Abstract
In the last 20 years, MIMO wireless communication has gone from concept to commercial deployments in millions of devices. Two flavors of MIMO widely researched in the Signal Processing community -- massive and mmWave -- are key components of 5G. In this talk, I will explain why MIMO communication has remained such a vibrant topic of signal processing research especially as systems have gone to millimeter wave and higher carrier frequencies. Then I will speculate on several directions for future MIMO research. I will talk about how other advancements in circuits, antennas, and materials may change the models and assumptions that are used in MIMO signal processing, leading to new algorithms and signal processing developments in the future.
Biography
Robert W. Heath Jr. is a Distinguished Professor in the Department of Electrical and Computer Engineering at the North Carolina State University (NC State). He is co-developer of the 6GNC initiative on next generation cellular communications at NC State. He is also the President and CEO of MIMO Wireless Inc. He is a Highly Cited Researcher who has published broadly in the areas of signal processing for wireless communications, with an emphasis on MIMO wireless communication systems for 4G, 5G and 6G cellular communication systems. His recent work includes algorithms for millimeter wave MIMO communications, circuit-aware communication theory, joint communication and radar, V2X, and machine learning for wireless communications. He authored “Introduction to Wireless Digital Communication” and co-authored “Millimeter Wave Wireless Communications” and “Foundations of MIMO Communications.”
Prof. Heath is a recipient of several awards including the 2012 Signal Processing Magazine Best Paper award, a 2013 Signal Processing Society best paper award, the 2014 EURASIP Journal on Advances in Signal Processing best paper award, and the 2014 Journal of Communications and Networks best paper award, the 2016 IEEE Communications Society Fred W. Ellersick Prize, the 2016 IEEE Communications Society and Information Theory Society Joint Paper Award, 2017 IEEE Marconi Prize Paper Award, the 2017 EURASIP Technical Achievement Award, the 2019 IEEE Communications Society Stephen O. Rice Prize, the 2019 IEEE Kiyo Tomiyasu Award, and the 2020 IEEE Signal Processing Society Donald G. Fink Overview Paper Award. He was EIC of IEEE Signal Processing Magazine from 2018-2020. He is a current member-at-large of the IEEE Communications Society Board-of-Governors (2020-2022) and a past member-at-large on the IEEE Signal Processing Society Board-of-Governors (2016-2018). He is a licensed Amateur Radio Operator, a registered Professional Engineer in Texas, a Private Pilot, a Fellow of the National Academy of Inventors, and a Fellow of the IEEE.