Expert Sessions
Tue, 24 May, 05:00 - 06:00 UTC
Karlheinz Brandenburg, Technische Universität Ilmenau, Germany and CEO of Brandenburg Labs GmbH
Chair: Woon-Seng Gan, Nanyang Technological University, Singapore
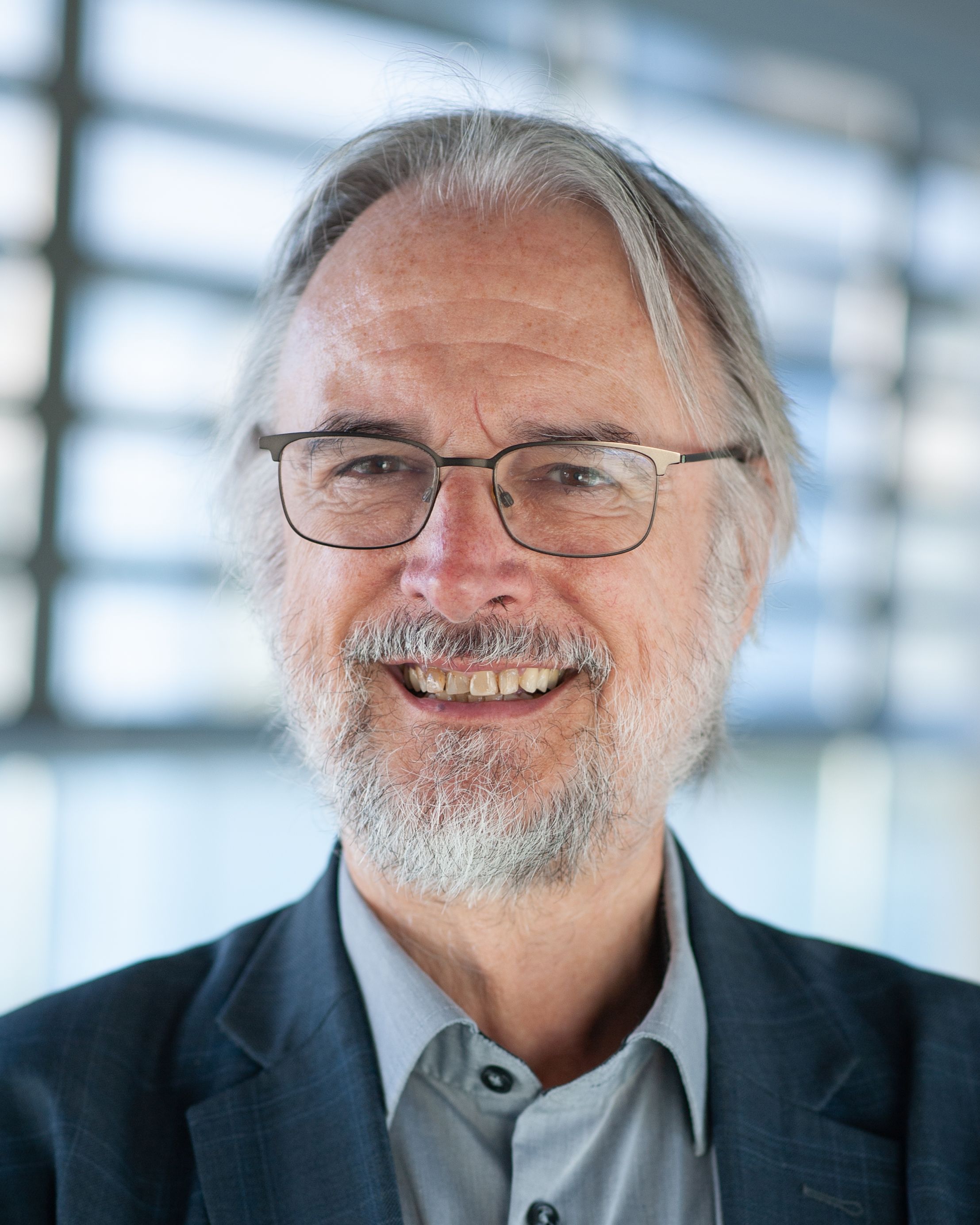
Since more than 40 years researchers have tried to deliver truly immersive sound via headphones. Until very recently, all the proposals to deliver spatial audio via headphones have fallen short of their promises.
Basic research at TU Ilmenau and other universities has given us a deeper insight into how the ears and the brain work while we are listening to sounds in a room. These results brought a massive improvement to binaural reproduction methods.
The talk will give a short overview over the techniques which have been used in the last decades. It will elaborate on all the cues used to improve listening with headphones including HRTF (Head Related Transfer Functions), BRIR (Binaural Room Impulse Responses) and room simulation techniques. It will then discuss quality parameters for binaural listening - including externalization of sounds. The emphasis will be on improved techniques (current state of the art) and how these will enable much better spatial reproduction.
Future concepts of headphones will incorporate additional sensors to track the head and body position of listeners. Together with knowledge about the room the listener is in, this enables a much more plausible reproduction of virtual sound sources. In conclusion this makes true Auditory Augmented Reality applications possible.
The talk will shed a light on the different technical issues which still have to be solved to sell consumer headphones which enable immersive sound.
Finally, future products by Brandenburg Labs GmbH will be presented. Among them is the vision of PARty (Personalized Auditory Reality). These devices will combine truly transparent audio (you don’t even realize you got headphones on your ear) with selective noise cancelling. They can bring in amplified real and virtual sound sources, all adapted to your current environment. The idea is to improve and personalize hearing for everybody even in difficult noisy environments like a party.
Karlheinz Brandenburg (Fellow IEEE SPS) received the Dipl.-Ing. and Dipl.-Math. degrees in electrical engineering and mathematics and the Dr.-Ing. degree in electrical engineering from the Friedrich-Alexander-Universität, Erlangen-Nürnberg, Germany,. He is currently Senior Professor (emeritus) at Technische Universität Ilmenau, Germany and CEO of Brandenburg Labs GmbH, a startup company specializing in immersive audio technologies.
Following times as a Postdoctoral Member of Technical Staff at AT&T Bell Laboratories in Murray Hill, U.S.A. and again Friedrich-Alexander-Universität he joined the Fraunhofer Institute for Integrated Circuits IIS, Erlangen, as head of the Audio and Multimedia Department. He is the founding director of the Fraunhofer-Institut für Digitale Medientechnologie (Fraunhofer IDMT), Ilmenau where he retired in July, 2019. For his pioneering work in digital audio coding (as a main contributor to the mp3 and AAC audio coding standards), perceptual measurement techniques, wave field synthesis, psychoacoustics, and analysis of audio and video signals he received many awards. Among them are the IEEE Masaru Ibuka Consumer Electronics Award, the German Future Award (shared with his colleagues), and the Audio Engineering Society Silver Medal Award. Furthermore, he is a member of the Hall of Fame of the Internet Society and the IEEE Consumer Electronics Association.
Tue, 24 May, 08:00 - 09:00 UTC
Yonina Eldar, Weizmann Institute of Science, Rehovot, Israel
Chair: Kai-Kuang Ma, Nanyang Technological University, Singapore
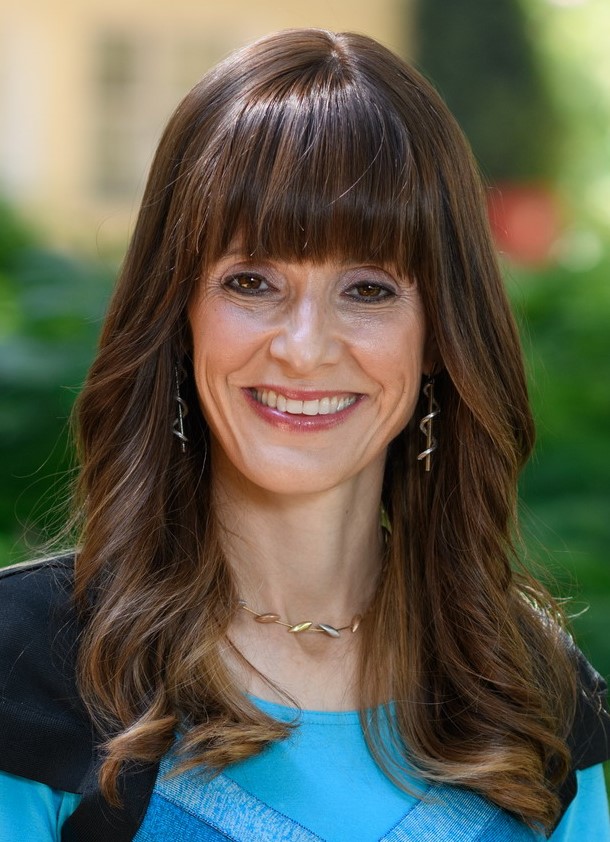
Deep neural networks provide unprecedented performance gains in many real-world problems in signal and image processing. Despite these gains, the future development and practical deployment of deep networks are hindered by their black-box nature, i.e., a lack of interpretability and the need for very large training sets.
On the other hand, signal processing and communications have traditionally relied on classical statistical modeling techniques that utilize mathematical formulations representing the underlying physics, prior information and additional domain knowledge. Simple classical models are useful but sensitive to inaccuracies and may lead to poor performance when real systems display complex or dynamic behavior. Here we introduce various approaches to model based learning which merge parametric models with optimization tools and classical algorithms leading to efficient, interpretable networks from reasonably sized training sets. We will consider examples of such model-based deep networks to image deblurring, image separation, super resolution in ultrasound and microscopy, efficient communication systems, and finally we will see how model-based methods can also be used for efficient diagnosis of COVID19 using X-ray and ultrasound.
Yonina Eldar is a Professor in the Department of Mathematics and Computer Science, Weizmann Institute of Science, Rehovot, Israel, where the heads the center for biomedical engineering. She is also a Visiting Professor at MIT, a Visiting Scientist at the Broad Institute, and an Adjunct Professor at Duke University and was a Visiting Professor at Stanford. She is a member of the Israel Academy of Sciences and Humanities, an IEEE Fellow and a EURASIP Fellow. She has received many awards for excellence in research and teaching, including the IEEE Signal Processing Society Technical Achievement Award (2013), the IEEE/AESS Fred Nathanson Memorial Radar Award (2014) and the IEEE Kiyo Tomiyasu Award (2016). She was a Horev Fellow of the Leaders in Science and Technology program at the Technion and an Alon Fellow. She received the Michael Bruno Memorial Award from the Rothschild Foundation, the Weizmann Prize for Exact Sciences, the Wolf Foundation Krill Prize for Excellence in Scientific Research, the Henry Taub Prize for Excellence in Research (twice), the Hershel Rich Innovation Award (three times), the Award for Women with Distinguished Contributions, and several teaching awards. She was selected as one of the 50 most influential women in Israel, and was a member of the Israel Committee for Higher Education. She is the Editor in Chief of Foundations and Trends in Signal Processing and heads the committee for Gender Fairness in Higher Education Institutions in Israel.
Wed, 25 May, 00:30 - 01:30 UTC
Roozbeh Jafari, Texas A&M University, USA
Chair: Saeid Sanei, Nottingham Trent University, UK.
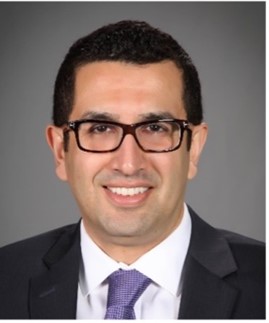
The bold vision of pervasive physiological monitoring, through proliferation of off-the-shelf wearables that began a decade ago, has created immense opportunities for precision medicine outside clinics and in ambulatory settings. Although significant progress has been made, several unmet needs remain; Lack of availability of advanced wearable sensing paradigms, noisy wearable data and labels in ambulatory settings, the unknown circumstances surrounding data capture in wearable paradigms, heterogeneity of the users both in terms of physiological and behavioral states, and often limited view into the user’s physiological state prevent extraction of actionable information.
This seminar presents several topics that coherently articulate on the vision and the opportunities of practicing “medicine in the wild” using wearables. We will present advanced wearable sensing paradigms including cuffless blood pressure monitoring using a miniaturized array of bio-impedance sensors combined with AI-assisted calibration-free techniques to extract blood pressure with clinical grade accuracy. In addition, we will explore the need for generalizable and customizable models resembling digital twin paradigms that can assist with such measurements and information extraction. The nature of noise on various wearable data modalities will be discussed and several signal processing techniques to address the noise, including particle filters and combinatorial algorithms will be discussed. Probabilistic and context-aware machine learning algorithms that can further combat the issue of noise and external undesirable confounders will be presented. These algorithms are well-suited to identify minor and yet important trends correlated with the user’s physiological states. Lastly, we will present personalization techniques at the level of data and machine learning/AI, that will enhance the ability to extract actionable information in the context of several real-world applications.
Digital health and wearables will play a significant role in the future of medicine outside clinics. The future directions present opportunities both in short-term translational research efforts with direct influence on clinical practice as well as long-term foundational development of theories and computational frameworks combining human physiology, physics, computer science, engineering, and medicine, all aimed at impacting the health and wellbeing of our communities.
Roozbeh Jafari (http://jafari.tamu.edu) is the Tim and Amy Leach Professor of Biomedical Engineering, Computer Science and Engineering and Electrical and Computer Engineering at Texas A&M University. He received his Ph.D. in Computer Science from UCLA and completed a postdoctoral fellowship at UC-Berkeley. His research interest lies in the area of wearable computer design and signal processing. He has raised more than $86M for research with $23M directed towards his lab. His research has been funded by the NSF, NIH, DoD (TATRC), DTRA, DIU, AFRL, AFOSR, DARPA, SRC and industry (Texas Instruments, Tektronix, Samsung & Telecom Italia). He has published over 200 papers in refereed journals and conferences. He has served as the general chair and technical program committee chair for several flagship conferences in the areas of wearable computers. Dr. Jafari is the recipient of the NSF CAREER award (2012), IEEE Real-Time & Embedded Technology & Applications Symposium best paper award (2011), Andrew P. Sage best transactions paper award (2014), ACM Transactions on Embedded Computing Systems best paper award (2019), and the outstanding engineering contribution award from the College of Engineering at Texas A&M (2019). He has been named Texas A&M Presidential Fellow (2019). He serves on the editorial board for the IEEE Transactions on Biomedical Circuits and Systems, IEEE Sensors Journal, IEEE Internet of Things Journal, IEEE Journal of Biomedical and Health Informatics, IEEE Open Journal of Engineering in Medicine and Biology and ACM Transactions on Computing for Healthcare. He is currently the chair of the IEEE Wearable Biomedical Sensors and Systems Technical Committee (elected) as well the IEEE Applied Signal Processing Technical Committee (elected). He serves on scientific panels for funding agencies frequently, served as a standing member of the NIH Biomedical Computing and Health Informatics (BCHI) study section (2017-2021), and is the inaugural chair of the NIH Clinical Informatics and Digital Health (CIDH) study section (2020-2022). He is a Fellow of the American Institute for Medical and Biological Engineering (AIMBE).
Wed, 25 May, 05:00 - 06:00 UTC
Zheng-Hua Tan, Aalborg University, Denmark
Chair: Ngai-Man (Man) Cheung, Singapore University of Technology and Design
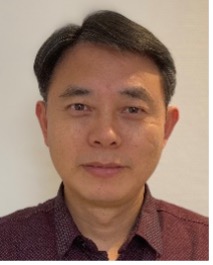
Humans learn much under supervision but even more without. Such will apply to machines. Self-supervised learning is paving the way by leveraging unlabeled data which is vastly available. In this emerging learning paradigm, deep representation models are trained by supervised learning with supervisory signals (i.e., training targets) derived automatically from unlabeled data itself. It is abundantly clear that such a learnt representation can be useful for a broad spectrum of downstream tasks.
As in supervised learning, key considerations in devising self-supervised learning methods include training targets and loss functions. The difference is that training targets for self-supervised learning are not pre-defined and greatly dependent on the choice of pretext tasks. This leads to a variety of novel training targets and their corresponding loss functions. This talk aims to provide an overview of training targets and loss functions developed in the domains of speech, vision and text. Further, we will discuss some open questions, e.g., transferability, and under-explored problems, e.g., learning across modalities. For example, the pretext tasks, and thus the training targets, can be drastically distant from the downstream tasks. This raises the questions like how transferrable the learnt representations are and how to choose training targets and representations.
Zheng-Hua Tan is a Professor in the Department of Electronic Systems and a Co-Head of the Centre for Acoustic Signal Processing Research at Aalborg University, Aalborg, Denmark. He is also a Co-Lead of the Pioneer Centre for AI, Denmark. He was a Visiting Scientist at the Computer Science and Artificial Intelligence Laboratory, MIT, Cambridge, USA, an Associate Professor at the Department of Electronic Engineering, Shanghai Jiao Tong University, Shanghai, China, and a postdoctoral fellow at the AI Laboratory, KAIST, Daejeon, Korea. His research interests are centred around deep representation learning and generally include machine learning, deep learning, speech and speaker recognition, noise-robust speech processing, and multimodal signal processing. He is the Chair of the IEEE Signal Processing Society Machine Learning for Signal Processing Technical Committee (MLSP TC). He is an Associate Editor for the IEEE/ACM TRANSACTIONS ON AUDIO, SPEECH AND LANGUAGE PROCESSING. He has served as an Associate/Guest Editor for several other journals. He was the General Chair for IEEE MLSP 2018 and a TPC Co-Chair for IEEE SLT 2016.
Wed, 25 May, 08:00 - 09:00 UTC
Shrikanth (Shri) Narayanan, University of Southern California, USA
Chair: Eliathamby Ambikairajah, University of New South Wales, Australia
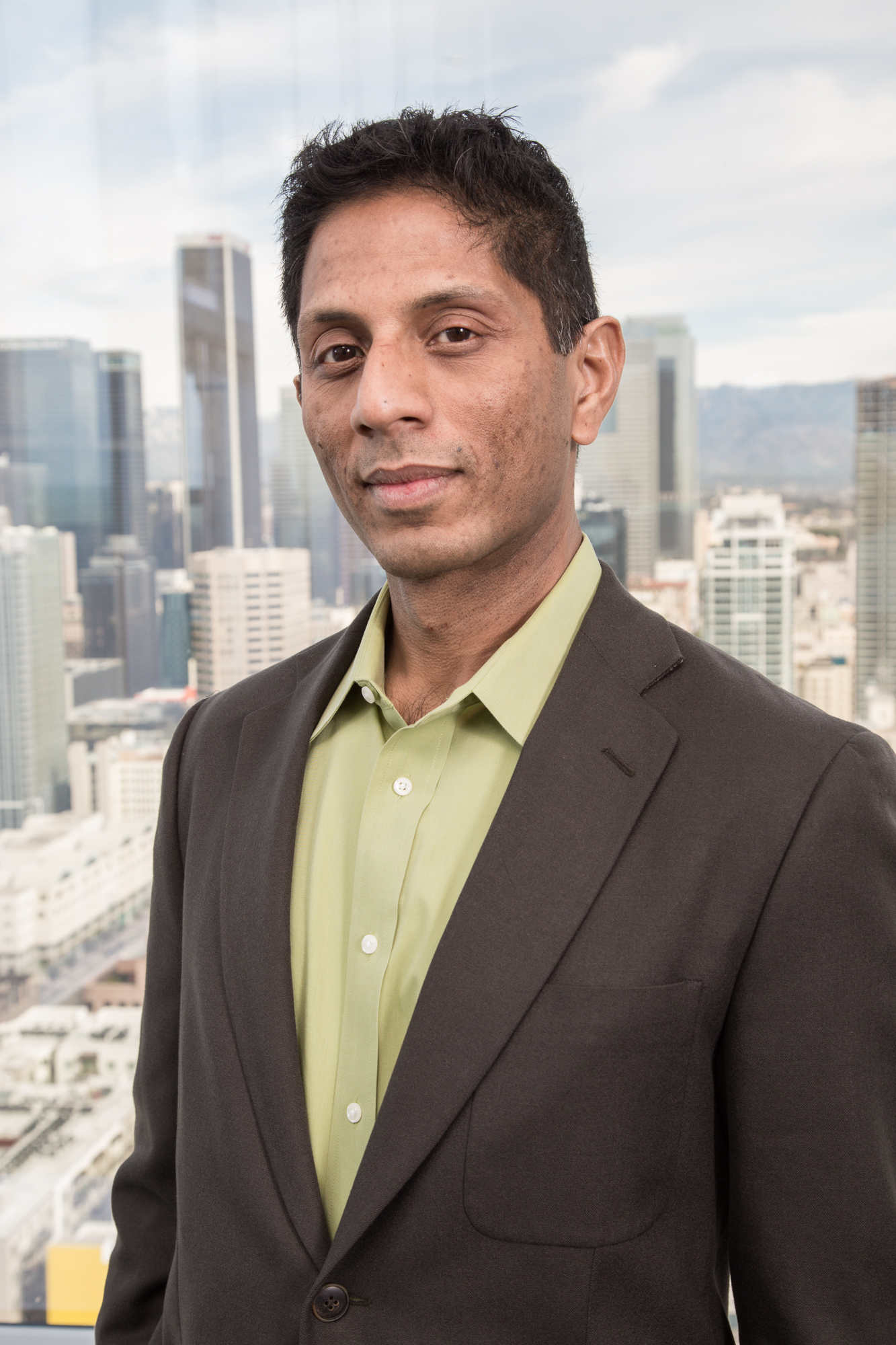
Converging developments across the machine intelligence ecosystem, from sensing and computing to data sciences, are enabling new human-centered technology applications in domains ranging from health and defense to education and media arts. A critical aspect of this endeavor requires addressing two intertwined challenges: understanding and illuminating the rich diversity across people and contexts and creating trustworthy technologies that work for everyone. This talk will highlight, with exemplary use cases of bio-behavior sensing and inference for predicting human physical and psychological states (e.g., health status, stress levels), the challenges and opportunities in creating trustworthy signal processing and machine learning approaches that are inclusive, equitable, robust, safe and secure e.g., with respect to protected variables such as gender/race/age/ability etc.
Shrikanth (Shri) Narayanan is University Professor and Niki & C. L. Max Nikias Chair in Engineering at the University of Southern California, where he is Professor of Electrical & Computer Engineering, Computer Science, Linguistics, Psychology, Neuroscience, Pediatrics, and Otolaryngology—Head & Neck Surgery, Director of the Ming Hsieh Institute and Research Director of the Information Sciences Institute. Prior to USC he was with AT&T Bell Labs and AT&T Research. He is a Fellow of the National Academy of Inventors, the Acoustical Society of America, IEEE, ISCA, the American Association for the Advancement of Science, the Association for Psychological Science, and the American Institute for Medical and Biological Engineering. He is presently VP for Education for the IEEE Signal Processing Society. He has received several honors including the 2015 Engineers Council’s Distinguished Educator Award, a Mellon award for mentoring excellence, the 2005 and 2009 Best Transactions Paper awards from the IEEE Signal Processing Society and serving as its Distinguished Lecturer for 2010-11, a 2018 ISCA CSL Best Journal Paper award, and serving as an ISCA Distinguished Lecturer for 2015-16, Willard R. Zemlin Memorial Lecturer for ASHA in 2017, and the Ten Year Technical Impact Award in 2014 and the Sustained Accomplishment Award in 2020 from ACM ICMI. He has published over 900 papers and has been granted eighteen U.S. patents.
Thu, 26 May, 05:00 - 06:00 UTC
Hung-yi Lee, National Taiwan University, Taiwan
Chair: Ville Hautamaki, National University of Singapore
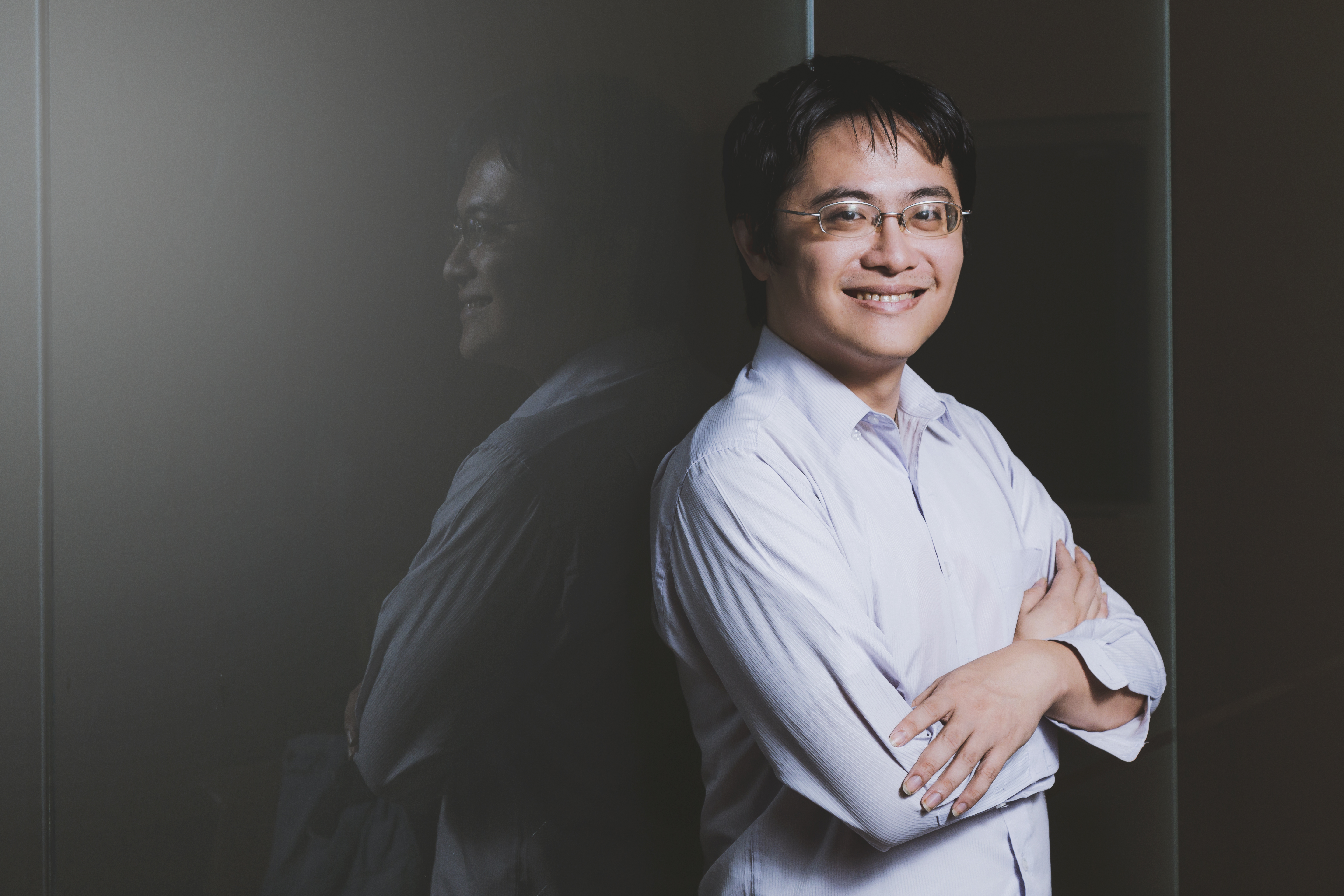
Self-supervised learning (SSL) has shown to be vital for advancing research in natural language processing (NLP), computer vision (CV), and speech processing. The paradigm pre-trains a shared model on large volumes of unlabeled data and achieves state-of-the-art for various tasks with minimal adaptation. This talk will share some interesting findings from the SSL models. For example, why do SSL models like BERT perform so well on NLP tasks? Generally, BERT is considered powerful in NLP because it can learn the semantics of words from large amounts of text data. Is this real? This talk will showcase some recent findings on the interdisciplinary capabilities of the SSL models that will change the way you think about the SSL models. This talk has little overlap with the ICASSP 2022 tutorial "Self-supervised Representation Learning for Speech Processing".
Hung-yi Lee is an associate professor of the Department of Electrical Engineering of National Taiwan University (NTU), with a joint appointment at the Department of Computer Science & Information Engineering of the university. His recent research focuses on developing technology that can reduce the requirement of annotated data for speech processing (including voice conversion and speech recognition) and natural language processing (including abstractive summarization and question answering). He won Salesforce Research Deep Learning Grant in 2019, AWS ML Research Award in 2020, Outstanding Young Engineer Award from The Chinese Institute of Electrical Engineering in 2018, Young Scholar Innovation Award from Foundation for the Advancement of Outstanding Scholarship in 2019, Ta-You Wu Memorial Award from Ministry of Science and Technology of Taiwan in 2019, and The 59th Ten Outstanding Young Person Award in Science and Technology Research & Development of Taiwan. He owns a YouTube channel teaching deep learning in Mandarin with about 100k Subscribers.
Thu, 26 May, 06:00 - 07:00 UTC
Akihiko K. Sugiyama, Yahoo! JAPAN Research, Tokyo, Japan
Chair: Andy Khong, Nanyang Technological University, Singapore
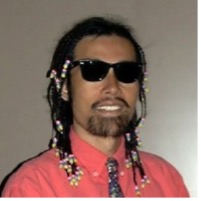
This talk presents an overview of patents from a technical and business point of view with an emphasis on the value. Patents are defined by the law in many countries to protect the rights of the inventor. Patent application documents take a special form which is often difficult to read and write for non-experts. Through explanation of items in the patent application documents, how to make your patent stronger and profitable in business is presented. A patent lawsuit example and some actual application documents make the talk easier to understand. Although patent attorneys generally undertake the drafting process, the audience learns that serious commitment of the inventor to drafting a patent is essential to strong and profitable patents.
Akihiko Sugiyama (a.k.a. Ken Sugiyama), affiliated with Yahoo! JAPAN Research, has been engaged in a wide variety of research projects in signal processing such as audio coding and interference/noise control. Prior to Yahoo Japan, he had a long career at NEC Central Research Laboratories as a research engineer. He served as the Chair of Audio and Acoustic Signal Processing Technical Committee, Signal Processing Society (SPS), as associate editor for Trans. Signal Processing, as the Secretary and a Member at Large to the SPS Conference Board, as a member of the SPS Awards Board, as the Chair of SPS Japan Chapter, and a member of IEEE Fellow Committee. He was a Technical Program Chair for ICASSP2012. Currently, he serves as a member of the IEEE Fellow Committee and the IEEE James Clerk Maxwell Medal Committee. He has contributed to 17 chapters of books and is the inventor of 217 registered patents with more pending applications in the field of signal processing. He received 20 awards such as the 2002 IEICE Best Paper Award, the 2006 and 2018 IEICE Achievement Award, the 2013 Ichimura Industry Award, and the 2021 IEICE Distinguished Achievement and Contribution Award. He has delivered 167 invited talks in 87 cities of 30 countries. He is a past SPS Distinguished Industry Speaker, a Renowned Distinguished Speaker (The Rock Star) for Consumer Technology Society (CTS) and a past Distinguished Lecturer for SPS and CTS.
Thu, 26 May, 09:00 - 10:00 UTC
1Catarina Botelho and 2Ayimnisagul Ablimit,
1Instituto Superior Técnico, Portugal; 2Universität Bremen, Germany
Chair: Tanja Schultz, University of Bremen, Germany
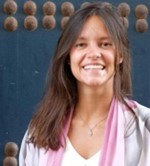
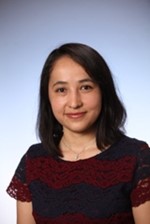
Today’s overburdened health systems worldwide face numerous challenges, aggravated by an increased aging population. Speech emerges as a rich, and ubiquitous biomarker with strong potential for the development of low-cost, widespread, and remote casual testing tools for several diseases. In fact, speech encodes information about a plethora of diseases, which go beyond the so-called speech and language disorders, and include neurodegenerative diseases, mood and anxiety-related diseases, and diseases that concern respiratory organs.
Recent advances in speech processing and machine learning have enabled the automatic detection of these diseases. Despite exciting results, this active research area faces several challenges that arise mostly from the limitations of the current datasets. They are typically very small, obtained in very specific recording conditions, for a single language, and concerning a single disease.
These challenges provide the guidelines for our research: how to deal with data scarcity? How to disentangle the effects of aging or other coexisting diseases in small, cross sectional datasets? How to deal with changing recording conditions, namely across longitudinal studies? How to transfer results across different corpora, often in different languages? Can other modalities (e.g. visual speech, EMG) provide complementary information to the acoustic speech signal? Are the results generalizable, explainable and fair?
In this talk, we will illustrate these challenges for different diseases, in particular with our work on the detection of Alzheimer’s disease in the context of longitudinal corpus and cross corpora analysis. We will also explore multimodal approaches for the prediction of obstructive sleep apnea.
Catarina Botelho is a PhD student at Instituto Superior Técnico / INESC-ID, Universidade de Lisboa since 2019. Her research topic is "Speech as a biomarker for speech affecting diseases”, focusing on the use of speech for medical diagnosis, monitoring and therapy. Particularly, she worked with obstructive sleep apnea, Parkinson's Disease, Alzheimer’s Disease, and COVID-19, and multimodal signals including EMG and visual speech. She was a research intern at Google AI, Toronto. She has been involved in the student advisory committee of the International Speech Communication Association (ISCA-SAC), since 2020, and currently acts as General Coordinator. She is also an IEEE student member.
Ayimnisagul Ablimit received her master’s degree in computer science from the Universität Bremen, Germany and she is a PhD student at Cognitive Systems Lab, Universität Bremen since July 2019. Her research topic is “speech-based cognitive impairment screening”, focusing on developing automatic speech recognition systems for spontaneous speech corpora, developing multilingual automatic speech recognition systems, conversational speech-based Alzheimer’s Disease, and Age-Associated Cognitive Decline screening, speech-based cognitive performance detection. She has been an IEEE Student Member since March 2019.
Fri, 27 May, 00:30 - 01:30 UTC
Xavier Bresson, National University of Singapore
Chair: Kai-Kuang Ma, Nanyang Technological University, Singapore
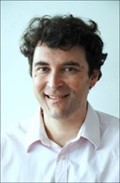
In the past years, deep learning methods have achieved unprecedented performance on a broad range of problems in various fields from computer vision to speech recognition. So far research has mainly focused on developing deep learning methods for grid-structured data, while many important applications have to deal with graph structured data. Such geometric data are becoming increasingly important in computer graphics and 3D vision, sensor networks, drug design, biomedicine, recommendation systems, NLP and computer vision with knowledge graphs, and web applications. The purpose of this talk is to introduce convolutional neural networks on graphs, as well as applications of these new learning techniques.
Xavier Bresson is an Associate Professor in the Department of Computer Science at the National University of Singapore (NUS). His research focuses on Graph Deep Learning, a new framework that combines graph theory and neural network techniques to tackle complex data domains. In 2016, he received the US$2.5M NRF Fellowship, the largest individual grant in Singapore, to develop this new framework. He was also awarded several research grants in the U.S. and Hong Kong. He co-authored one of the most cited works in this field (10th most cited paper at NeurIPS), and he has recently introduced with Yoshua Bengio a benchmark that evaluates graph neural network architectures. He has organized several workshops and tutorials on graph deep learning such as the recent IPAM'21 workshop on "Deep Learning and Combinatorial Optimization", the MLSys'21 workshop on "Graph Neural Networks and Systems", the IPAM'19 and IPAM'18 workshops on "New Deep Learning Techniques", and the NeurIPS'17, CVPR'17 and SIAM'18 tutorials on "Geometric Deep Learning on Graphs and Manifolds". He has been a regular invited speaker at universities and companies to share his work. He has also been a speaker at the KDD'21, AAAI'21 and ICML'20 workshops on "Graph Representation Learning", and the ICLR'20 workshop on "Deep Neural Models and Differential Equations". He has taught graduate courses on Deep Learning and Graph Neural Networks at NUS, and as a guest lecturer for Yann LeCun's course at NYU. Twitter: https://twitter.com/xbresson, Scholar: https://scholar.google.com.sg/citations?hl=en&user=9pSK04MAAAAJ, GitHub: https://github.com/xbresson, LinkedIn: https://www.linkedin.com/in/xavier-bresson-738585b
Fri, 27 May, 05:00 - 06:00 UTC
Emmanuel Vincent, Inria Nancy - Grand Est, France
Chair: Rohan Kumar Das, Fortemedia, Singapore
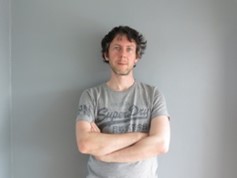
Large-scale collection, storage, and processing of speech data poses severe privacy threats. Indeed, speech encapsulates a wealth of personal data (e.g., age and gender, ethnic origin, personality traits, health and socio-economic status, etc.) which can be linked to the speaker's identity via metadata or via automatic speaker recognition. Speech data may also be used for voice spoofing using voice cloning software. With firm backing by privacy legislations such as the European general data protection regulation (GDPR), several initiatives are emerging to develop privacy preservation solutions for speech technology. This talk focuses on voice anonymization, that is the task of concealing the speaker's voice identity without degrading the utility of the data for downstream tasks. I will i) explain how to assess privacy and utility, ii) describe the two baselines of the VoicePrivacy 2020 and 2022 Challenges and complementary methods based on adversarial learning, differential privacy, or slicing, and iii) conclude by stating open questions for future research.
Emmanuel Vincent (SM'09, F'22) received the Ph.D. degree in music signal processing from Ircam in 2004 and joined Inria, the French national research institute for digital science and technology, in 2006. He is currently a Senior Research Scientist and the Head of Science of Inria Nancy - Grand Est. His research covers several speech and audio processing tasks, with a focus on privacy preservation, learning from little or no labeled data, source separation and speech enhancement, and robust speech and speaker recognition. He is a founder of the MIREX, SiSEC, CHiME, and VoicePrivacy challenge series. He is a scientific advisor of the startup company Nijta, which provides speech anonymization solutions.