Thu, 26 May, 05:00 - 06:00 UTC
Hung-yi Lee, National Taiwan University, Taiwan
Chair: Ville Hautamaki, National University of Singapore
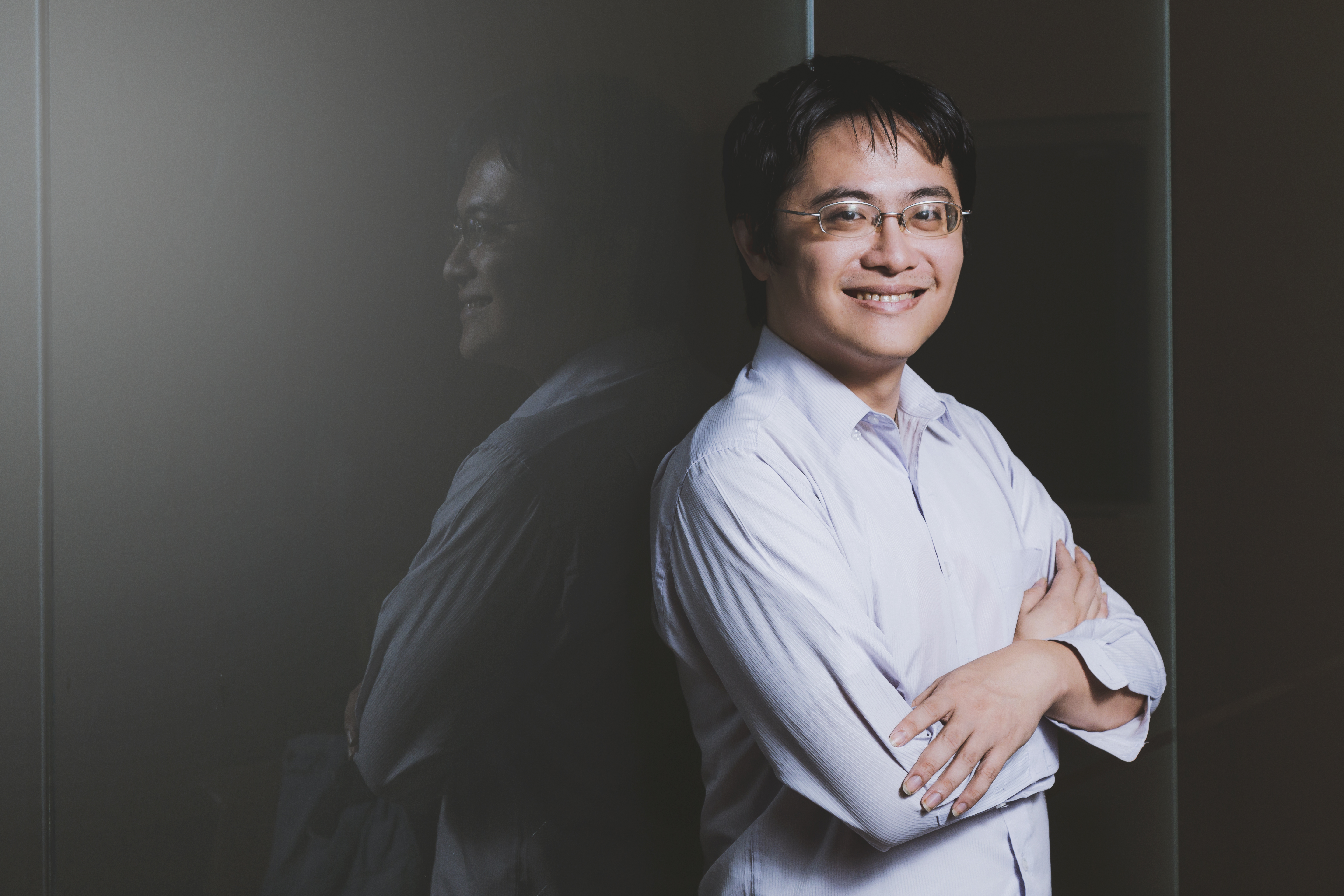
Self-supervised learning (SSL) has shown to be vital for advancing research in natural language processing (NLP), computer vision (CV), and speech processing. The paradigm pre-trains a shared model on large volumes of unlabeled data and achieves state-of-the-art for various tasks with minimal adaptation. This talk will share some interesting findings from the SSL models. For example, why do SSL models like BERT perform so well on NLP tasks? Generally, BERT is considered powerful in NLP because it can learn the semantics of words from large amounts of text data. Is this real? This talk will showcase some recent findings on the interdisciplinary capabilities of the SSL models that will change the way you think about the SSL models. This talk has little overlap with the ICASSP 2022 tutorial "Self-supervised Representation Learning for Speech Processing".
Hung-yi Lee is an associate professor of the Department of Electrical Engineering of National Taiwan University (NTU), with a joint appointment at the Department of Computer Science & Information Engineering of the university. His recent research focuses on developing technology that can reduce the requirement of annotated data for speech processing (including voice conversion and speech recognition) and natural language processing (including abstractive summarization and question answering). He won Salesforce Research Deep Learning Grant in 2019, AWS ML Research Award in 2020, Outstanding Young Engineer Award from The Chinese Institute of Electrical Engineering in 2018, Young Scholar Innovation Award from Foundation for the Advancement of Outstanding Scholarship in 2019, Ta-You Wu Memorial Award from Ministry of Science and Technology of Taiwan in 2019, and The 59th Ten Outstanding Young Person Award in Science and Technology Research & Development of Taiwan. He owns a YouTube channel teaching deep learning in Mandarin with about 100k Subscribers.